AI in Quality Assurance: Pioneering the Future of Automation
Unleash the power of AI in quality assurance. Explore pioneering automation strategies that elevate quality standards and streamline your operations

Aarushi Kushwaha, 2024-10-08
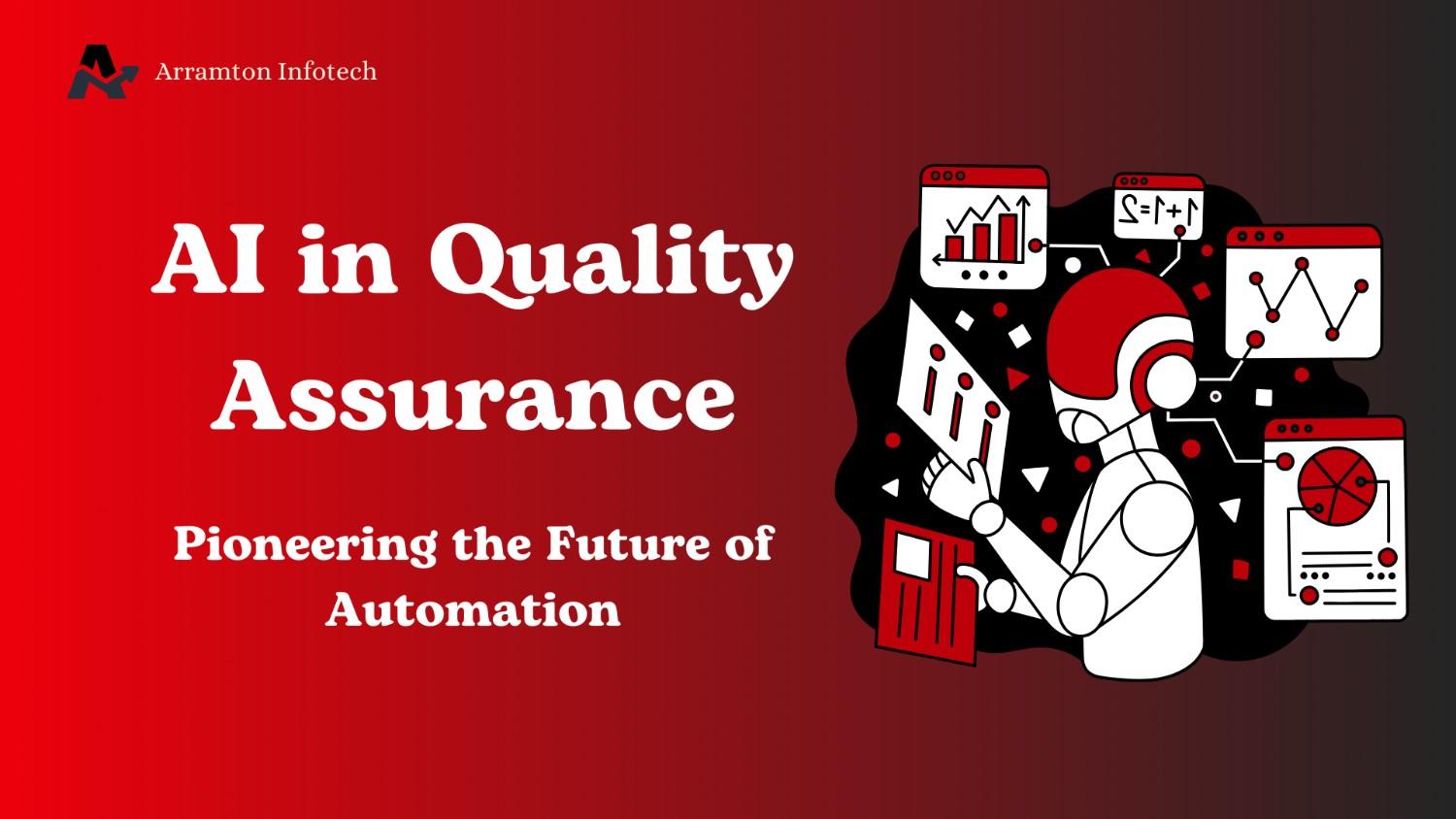
If you are a developer or a tech enthusiast, you will see how QA AI is revolutionizing software testing processes, making them faster and wiser.
Manual testing was labor-intensive and time-consuming, requiring significant effort to meet quality standards.
AI has revolutionized testing by automating the process and automatically generating test cases and data, among other things.
AI Applications in QA, Software Testing Quality assurance and testing through software are significantly affected by artificial intelligence.
AI offers several benefits that improve the testing process's efficiency and effectiveness.
Traditionally, QA relied heavily on manual testing methods, often tiring, time-consuming, and prone to human error.
In this article, we will discuss the role of AI quality assurance, its benefits, the popular tools and platforms for quality assurance AI, and the scope of AI QA in the future.
Table of Contents
- What is Quality Assurance?
- Quality Assurance and Management Methods: A Revolution
- The Role of AI Quality Assurance
- Advantages of QA AI Testing
- 1. Accelerated Timelines
- 2. Well Research Build Release
- 3. Effortless Test Planning
- 4. Role of Tester
- 5. Predictive Analysis
- 6. Effective Test Case Development
- 7. Efficient Regression Testing
- 8. Visual User Interface Testing
- 9. Enhanced Defect Tracing
- 11. Increased Test Coverage
- 12. Resourceful Bug Detection and Application Verification
- 13. Optimization of Resources
- Levels of QA Testing: Impact of AI on Quality Assurance
- Popular QA AI Tools
- Top AI Platforms
- The Future Scope of AI in Quality Assurance (QA)
- 1. Automated Test Case Generation: The Smart Way of Testing
- 2. Intelligent test scripting and maintenance: Adaptive Change
- 3. Predictive Analysis for Defect Prevention: Catching Bugs Before They Even Have a Chance to Begin
- 4. Efficient Test Execution and Prioritization: Focus on What Matters
- 5. Automated Bug Detection and Root Cause Analysis: Faster Problem Solving
- 6. NLP for Enhanced Understanding of Requirement
- 7. Optimization of Performance Testing-Building Stronger Software
- 8. Continuous Testing and Integration: Data-Driven QA AI
- 9. Behavioral Testing with AI-Testing from the User's Perspective
- 10. Security Testing Reinforcement: Cyber Threats vs. AI
- Conclusion
- Frequently Asked Questions
What is Quality Assurance?
Quality Assurance refers to the overall set of processes and methodologies developed for ensuring compliance with the standard and requirement prerequisites in the processes and products.
This involves intensive test processes, surveillance, and continuous improvement of overall quality.
However, the evolving QA AI technologies have dramatically changed the methodologies, providing unprecedented automation, precision, and scalability.
The evolution might positively influence AI’s impact on quantum computation. Artificial intelligence keeps stepping into various industries and automates multiple tasks.
Quality Assurance with AI is another addition.
Quality Assurance and Management Methods: A Revolution
Quality assurance strategies have changed significantly today. Those existing in the 1980s have strived to meet the software development, delivery approaches, and cycle requirements.
The current case, termed continuous testing, is dominated by the Agile and CI/CD approaches.
Although termed one of the most advanced levels of software testing using artificial intelligence, continuous testing holds with it a few critical challenges in quality assurance workflow procedures:
» Siloed automation
» Lack of end-to-end visibility of the requirements
» The high volume of the test
The industry must transition to autonomous testing that guarantees zero-touch QA to resolve these challenges.
Recommendation: Unleashing the Potential of Artificial Intelligence in the Oil and Gas Industry: 10 Use Cases, Benefits, and Examples
The Role of AI Quality Assurance
Testing software is usually time-consuming. The testing requires meticulous preparation to generate relevant test data.
Manual testing is prone to human errors, leading to higher costs and extended timelines, a significant concern for organizations.
Further, with an increase in the size of the software, the tests also increase, making the maintenance of an extensive test process difficult and achieving proper code coverage challenging.
Artificial intelligence has a record of improvising traditional tasks with more efficiency and productivity, for example, its contribution to quantum computing methods.
If we talk of the test cases of QA AI, here are the ways testers are using AI presently:
» AI Implementation through Image-based Testing
» Determination of whether to run a test script or not
» API testing monitoring
» Automation of tasks
AI plays several critical roles in quality assurance, such as:
1. Test Automation
AI-based tools can automatically repeat tasks like test case generation, execution, and analysis.
AI algorithms learn from experience, enhancing test coverage, detecting risks, and significantly reducing manual testing time.
2. Comprehensive Coverage of Tests
With an intelligent generation of test scenarios, the AI develops extensive coverage. It automatically scans all diverse data combinations; thus, defects go unnoticed during manual testing.
3. Dynamic Test Case Generation
Using techniques like genetic algorithms and neural networks, AI dynamically creates new test cases as requirements and system behavior change.
The effect of continuous evolution in software creates fresh requirements and conditions and is adapted to test suites to maintain effectiveness.
4. Defect Prediction and Prevention
AI analyzes code changes and project data, predicting potential defects before they occur.
Proactive QA enables teams to catch risky areas early to create quality software with fewer lines of code or less rework.
5. Natural Language Processing (NLP)
AI-based tools that leverage NLP can enhance accuracy and consistency by scanning text for errors and compliance. This works well for text-heavy industries like software documentation and customer support.
This also consolidates the textual content QA process, improving efficiency and quality standards.
6. Performance Testing Optimization
AI can imitate thousands of virtual users, testing performance under varying conditions to help QA teams identify restrictions and optimize scalability, ensuring a smooth user experience even under heavy loads.
7. Self-Healing Systems
AI makes it possible to have self-healing systems that will eventually detect and recover from failures during testing or even in production.
With AI-based monitoring and anomaly detection, businesses can minimize downtime and build more reliable systems.
Recommended Read: AI in CRM: Redefining How Businesses Connect with Customers
Advantages of QA AI Testing
AI brings numerous advantages to the QA and testing processes after the integration. It extends from speed to accuracy and efficiency.
Here are some significant benefits:
1. Accelerated Timelines
AI scans code extremely fast and can find many errors. It can do a task much longer for a human tester.
It doesn't get burnt out and is adaptive to changes in the code, recognizing whether new issues are features or bugs.
This can speed up the overall development process.
2. Well Research Build Release
AI helps companies analyze similar apps to understand what factors seem to make a difference between one and another.
Knowing the demands of the market, with the help of AI, it becomes easy to ensure that new software releases come out stable and with specific goals, reducing the chances of failures after release.
3. Effortless Test Planning
AI-powered QA tools can automatically plan test case scenarios by analyzing data and generating tests without human intervention.
Such tasks save time associated with planning and release the QA person from mundane tasks to focus on more complex tasks.
4. Role of Tester
QA AI requires a tester to learn new skills, like analysis of natural language processing (NLP), business intelligence, and algorithmic analysis.
This increases testing accuracy and enables testers to support the development process strategically.
5. Predictive Analysis
AI helps enable the AI QA teams to foresee the needs and behavior of users by analyzing their data.
This allows web and app developers to head over the market trends, and eventually, the support will be well provided and the products more user-friendly.
6. Effective Test Case Development
AI quickly generates better test cases by analyzing project data in seconds, offering deeper insights than traditional methods.
7. Efficient Regression Testing
Regression testing often becomes too complex to perform by hand.
AI can automate repetitive regression tests and detect even the slightest changes in the user interface, like changing color or modifying shape, which human testers might not detect.
8. Visual User Interface Testing
AI enhances UI testing by capturing various elements that are not workable to test via the manual layout or visual design process.
AI tools catch on minute details that a human may miss, enhancing the user's visual experience when accessing websites and applications.
9. Enhanced Defect Tracing
AI quickly and automatically detects bugs that traditional testing environments often miss.
AI also tracks duplicate errors and failure patterns through its findings, which makes it easier for the development team to fix issues while the application expands.
10. Continuous Integration and Delivery (CI/CD) Improvement
CI/CD pipelines can easily be integrated with AI so that all the testing of code and deploying activities get automated.
The CI/CD automatically tests every code change so that releases happen with speed and high reliability.
11. Increased Test Coverage
AI automatically generates rich test cases that significantly improve test coverage.
Edge case testing and rare functionalities will be assured, making the software effective and reliable.
12. Resourceful Bug Detection and Application Verification
AI tools are excellent for fast and accurate bug detection, as they help find any problem in its advanced stages by analyzing the code.
Hence, it accelerates fixing bugs, ensuring that applications are stable and reliable with all essential modules duly tested.
13. Optimization of Resources
AI tools can aid in the effective allocation of resources as, based on how critical and complex the testing task is, AI tools help prioritize such tasks.
Along with this, through the analysis of future requirements and needs, AI tools help to prepare better planning and resource management for it.
Explore Artificial intelligence impact on the Sports industry: AI in Sports: Redefining Performance, Strategy, and Engagement with Cutting-Edge Technology
Levels of QA Testing: Impact of AI on Quality Assurance
In the fast-paced tech world, it is now necessary for businesses to pay more attention to software products at higher quality levels.
Therefore, knowledge of the six stages of artificial intelligence integration in quality assurance (QA) testing is necessary.
Now, let's break them down one after the other:
Level Zero: Manual, Repetitive Testing
It's so unchanging at this introductory stage. For example, if one enters a field into a webpage, the addition applies to the test for that field.
Adding a form applies testing for all fields; after adding a new page, all components and forms compulsorily fall under testing again.
Overcomplicating the process with too many tests can lead to missed bugs or insufficient testing of the app's functionality.
To address this, failed tests should be reviewed to determine whether a bug or a new baseline expected behavior caused it.
Level One: AI-Assisted Testing
At this stage, AI is utilized to make QA more independent. Traditionally, AI systems analyzed the code structure (Document Object Model or DOM), but now they go further, visually evaluating page layouts.
This will assist in writing tests you would have had to do manually otherwise.
Modern AI technology can generate test scripts and automatically detect whether the test is a pass or fail.
If a test fails, it will raise an alert so you can immediately tell if it is a bug or an accident because of some changes to the software.
Level Two: Organization and Understanding Changes
Level two builds on that. AI can even test visual elements on a page without manually checking things.
However, reviewing every test failure remains labor-intensive work.
At level two, AI becomes more intelligent. It can sense changes within the app, making it seem as if it were a human.
For instance, it can logically group multiple pages with related changes. Then, it asks if such changes should be accepted or rejected altogether, saving time in processing.
Level Three: Independent AI Evaluation
Although human inspection is necessary at the initial stages, in stage three, AI starts to assume responsibility.
Applying machine learning algorithms can determine whether the application's visual design has a good UI/UX design.
At this stage, the AI can think for itself and independently analyze the pages by comprehending all design rules and data patterns.
That analysis reflects hundreds of results over time and spots essential changes based on machine learning input with less human intervention.
Level Four: AI-Driven Testing
At level four, AI starts running tests itself. Since AI understands the app just like humans, it can run tests based on user interactions and how the page flows.
With reinforcement learning, AI can watch how users interact with the app over time, visualize these, and use that knowledge to set up automated tests.
In other words, AI can now carry out tests without being given step-by-step instructions.
Level Five: Full AI Autonomy
That is a very science-fiction fantasy, but this is the future of AI in QA.
At level five, it's not only that the AI will understand the app, but it will also be able to respond to product managers while even running the testing process independently.
While most AI systems currently sit at level one, there are already applications of this in automation areas like visual UI testing, API testing, and spidering, essentially crawling through a website's structure.
Full autonomy might be far off, but the building blocks for such capability are falling into place today.
Popular Read: AI in Investing: How to Use Artificial Intelligence To Improve Your Investment Results
Popular QA AI Tools
» Testim
» Applitools
» TestCraft
» Function
Top AI Platforms
» IBM Watson
» Microsoft Azure AI
» Amazon SageMaker
» Google Cloud AI Platform
The Future Scope of AI in Quality Assurance (QA)
MarketsandMarkets estimates that the AI market will be massive by the end of 2030, when it's expected to reach $1,339.1 billion, compared to the current projected figures in 2024, which are around $214.6 billion.
This skyrocketing indicates how much AI will likely drive all the activities across sectors like QA.
The future advantages of AI in QA will emerge through automation because self-learning AI models continuously evolve when developing and executing test cases.
Here’s a look at some of the ways AI will transform QA in the future:
1. Automated Test Case Generation: The Smart Way of Testing
Test case creation is time-consuming as it might overlook other significant scenarios.
This will soon change with the rise of AI and ML algorithms, which will automatically generate test cases.
These intelligent algorithms will learn from the previous data while testing and automatically note down other critical scenarios overlooked in manual creation.
This will improve more towards better coverage and efficient testing processes.
2. Intelligent test scripting and maintenance: Adaptive Change
Maintaining the script in case a code change happens frequently is one of the most significant issues with test automation.
AI-powered tools will resolve this issue by generating brilliant scripts that adapt automatically to changes in the application code.
Updating a test script based on changes in the application development by machine learning tools will reduce the time spent on the maintenance of the script.
3. Predictive Analysis for Defect Prevention: Catching Bugs Before They Even Have a Chance to Begin
While it tries to identify and predict defects, AI will also prevent them.
Based on the patterns in the past defect data, it will be possible to detect prevailing signal risks with machine learning models.
This will enable the teams to start more early interventions to save time, maximize resource usage, and improve software quality.
4. Efficient Test Execution and Prioritization: Focus on What Matters
Firstly, you should optimize the testing procedures with AI-based algorithms to select the test cases based on their relevance and significant impact during appropriate functionalities.
Such algorithms, analyzing historical data and user behavior patterns, shall emphasize the most critical functions to deliver swift feedback, smoothening the testing process.
5. Automated Bug Detection and Root Cause Analysis: Faster Problem Solving
Quick detection and resolution of bugs make for effective software development.
Testing AI-based tools will automatically detect issues, and teams will quickly identify the root causes of the problems.
Debugging would be faster, and therefore, efficiency in the development process would be enhanced.
6. NLP for Enhanced Understanding of Requirement
Easy conversion of requirements into test cases- the NLP algorithms will help bridge this gap.
It will understand and extract vital information from textual requirements, making creating more accurate test cases accessible.
This will reduce the chasm of miscommunication and improve collaboration between development and QA AI teams.
7. Optimization of Performance Testing-Building Stronger Software
AI and ML would be game changers in the performance testing space.
So, all that was done in real-time by actual execution with specific parameterization could also be simulated and predicted how an application would perform under many given scenarios.
Machine learning algorithms would analyze the bottlenecks and suggest improvements, creating more robust, scalable software products.
8. Continuous Testing and Integration: Data-Driven QA AI
Adding AI to continuous testing and integration will accelerate feedback flow.
Automated analysis of test results and machine learning algorithms will help teams detect trends and patterns over time.
This creates a tight feedback loop that improves decision-making and fosters continuous improvement in software quality.
9. Behavioral Testing with AI-Testing from the User's Perspective
Apply outstanding AI-powered tools to imitate the way users interact with applications.
The tools will ensure that the applications successfully achieve their ends from an end-user's point of view by providing thorough real-world testing of user interfaces and experiences.
This method will ensure that it allows for an intuitive, smooth user experience regardless of the platforms or environments.
10. Security Testing Reinforcement: Cyber Threats vs. AI
Security vulnerabilities will be at the core of testing the system as software development increases complexity.
AI and ML-based methods ensure that possible risks and vulnerabilities in the code are detected to provide cyber security.
Machine learning models will learn from past data about the patterns that identify security risks to protect applications from cyber threats and losses of sensitive information.
Conclusion
In a nutshell, integrating QA AI will completely modify the outlook of software testing.
Automating all those tedious and time-consuming repetitive tasks helps achieve maximum accuracy and test coverage by minimizing the possibilities of human error.
AI in quality assurance does more than just better the current testing practices; it even opens new avenues of predictive analysis, dynamic test generation, and self-healing systems.
As technology evolves, so will testing processes, which are becoming more advanced and complex—such as behavioral testing from a user's perspective and enhanced security testing to counter cyber threats.
The future of QA undoubtedly is AI-powered, providing companies with the tools they need to keep ahead of the continually growing demands of the tech world.
With such innovation, businesses can better their development processes, reduce costs, and deliver better software products to their users.
Furthermore, for any AI/ML development assistance, contact Arramton Infotech, a leading development company, to get all you need for your business growth.
Frequently Asked Questions
Q. What is QA In AI?
Ans: AI for QA represents the application of algorithms with neural networks and more AI tools to enhance the process of testing software automatically. AI-based services for QA will allow for improved accuracy, efficiency, and speed at which bugs and issues are identified in a software application and can make the processes predictive, proactive, and adaptive. This also saves from various types of human errors and saves human testers time focusing on more complex and critical tasks.
Q. What are the challenges with AI quality assurance?
Ans: Ensuring the quality of AI systems may bring some problems. This Is because it mainly focuses on the quality and quantity of the data used in training and validation. AI needs large datasets to be operative, but poor data quality or outdated data can adversely affect the model.
Q. What are the benefits of AI-driven predictive analytics in defect detection?
Ans: AI-driven predictive analytics in defect detection offers several benefits:
» Early error detection
» Accurate data
» Optimization of resources
Empowering Businesses with Technology